
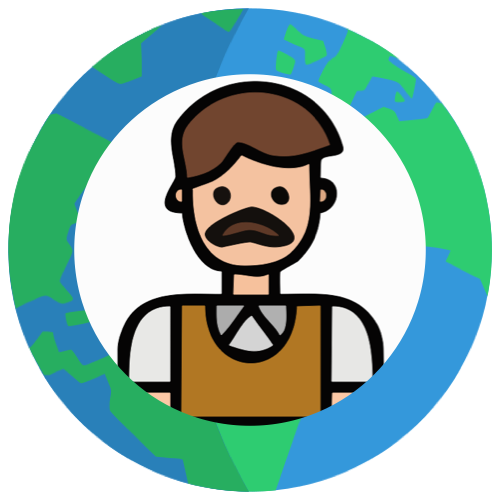
Once you get the pattern in your head it’s pretty easy to remember where to go. The tricky part is actually holding up your ring finger on its own.
Once you get the pattern in your head it’s pretty easy to remember where to go. The tricky part is actually holding up your ring finger on its own.
Base 10 on your hands is really base 1. Every finger is either 0 or 1 and we just count them! Base 12 we do have 12 positions each representing a digit, and two potential digits from our hands.
Binary is so much more efficient because you have 10 digits, just like in base 1, but you use them more efficiently.
The next logical step is trinary, if we can incorporate enough fingers it would go higher than binary. Wikipedia suggests three positions of your fingers - up, down, and somewhere in between, or folded - but I’d be surprised if anyone can realistically do that with all their fingers. However, using four fingers on each hand and pointing them at different knuckles/the tip of your thumb gets you 8 digits of base 4 (including not pointing at the thumb at all as 0)… And actually doesn’t tangle your fingers up too bad.
If only we could combine the two and get to 2^12… Sadly, this would require 12 thumbs.
Ooh, actually you can get to 2^8 without worrying about those pesky tendon issues by putting your fingertips against your thumb instead of trying to extend your fingers… Hmmm… Maybe we can even go to 2^10 this way by incorporating knuckles. Might lose some time today figuring out more hand counting systems. I wonder if anything higher than 2^10 is possible…
12 knuckles on your fingers, use your thumb to count em.
Binary wins though, learn to read it and you can count uo to 2^10. Well, sort of. Ring fingers and little fingers ruin it for me.
There are certainly blind dancers. Idk about martial arts but I wouldn’t be surprised.
Writing boring shit is LLM dream stuff. Especially tedious corpo shit. I have to write letters and such a lot, it makes it so much easier having a machine that can summarise material and write it in dry corporate language in 10 seconds. I already have to proof read my own writing, and there’s almost always 1 or 2 other approvers, so checking it for errors is no extra effort.
Newer laser can work on lighter hair, still less effective but possible nowadays.
What??
If you took all the racists and bigots in the world and put them in one country… It still wouldn’t be justified to wipe them out. I wouldn’t want to go to that country - it would certainly be among the worst places in the world - but I also wouldn’t suggest we invade and start murdering them.
And let’s be honest, not because they were fascists.
AI models don’t resynthesize their training data. They use their training data to determine parameters which enable them to predict a response to an input.
Consider a simple model (too simple to be called AI but really the underlying concepts are very similar) - a linear regression. In linear regression we produce a model which follows a straight line through the “middle” of our training data. We can then use this to predict values outside the range of the original data - albeit will less certainty about the likely error.
In the same way, an LLM can give answers to questions that were never asked in its training data - it’s not taking that data and shuffling it around, it’s synthesising an answer by predicting tokens. Also similarly, it does this less well the further outside the training data you go. Feed them the right gibberish and it doesn’t know how to respond. ChatGPT is very good at dealing with nonsense, but if you’ve ever worked with simpler LLMs you’ll know that typos can throw them off notably… They still respond OK, but things get weirder as they go.
Now it’s certainly true that (at least some) models were trained on CSAM, but it’s also definitely possible that a model that wasn’t could still produce sexual content featuring children. It’s training set need only contain enough disparate elements for it to correctly predict what the prompt is asking for. For example, if the training set contained images of children it will “know” what children look like, and if it contains pornography it will “know” what pornography looks like - conceivably it could mix these two together to produce generated CSAM. It will probably look odd, if I had to guess? Like LLMs struggling with typos, and regression models being unreliable outside their training range, image generation of something totally outside the training set is going to be a bit weird, but it will still work.
None of this is to defend generating AI CSAM, to be clear, just to say that it is possible to generate things that a model hasn’t “seen”.
Stealing from an individual is deplorable. I can understand why someone might want to respond aggressively (although to be clear I still don’t think it’s justified) if someone steals medication from an old lady… But from a shop?
It is normal, isn’t it? “Adult film actress” is a euphemism, I can’t imagine hearing anyone I know say it.
It’s interesting that when talking about films and TV we often use actor to describe both men and women, but in porn women are always actresses haha.
And BORU was actually interesting stories instead of… Compilations of other redditors’ creative writing.
I mean going vegetarian or vegan by definition reduces your options.
That said, there are lots of delicious slow cooked vegan foods. Despite what the other commenter says, there certainly are flavours & textures available that are not easily accessed on the hob, even eating plant based.
I have exams approaching so, yknow, in hell lmao.
I can’t blame them for asking for money, it’s a free app but it isn’t free to run.
That said the notifications are royally annoying. My partner has an android, but calls almost never come through and messages are hit and miss.
We have to get Half Life: Eli and Half Life: Dog first.
Yes, but that doesn’t mean they would be able to entirely make a new habitat habitable for them. You can’t dump a beaver in the Sahara and expect it to survive. Even if they did, like I said the impact on the preexisting ecosystem also needs to be managed or you just trade one problem for another.
You don’t have to do this in Botswana because the elephants are already in Botswana…
It’s called hackthebox not hackoutofthebox